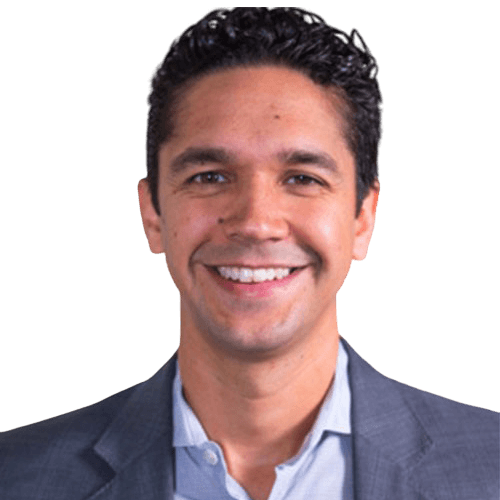
In our Ecosystem Explorer Series, we interview leaders from organizations who are advancing access to health data. Today’s interview is with Dr. Jason Bhan, Chief Medical Informatics Officer at Prognos.
Jason Bhan, MD, is a Family Physician and serves as the Chief Medical Officer at Prognos. He is regarded as a national expert in the applications of technology to healthcare and medicine, a topic on which he speaks regularly at institutions and conferences, such as Health 2.0, mHealth, New York’s eHealth Collaborative, and Health Datapalooza. He also has done extensive strategy consulting with pharmaceutical companies.
From 2007-2010, Dr. Bhan worked with Clinovations and managed several large hospital system EHR implementations, outcomes measurements and data analysis. Dr. Bhan obtained his Doctor of Medicine at the University of Miami School of Medicine and he is board certified in Family Medicine.
Prognos Health is a trusted provider of actionable real-world data (RWD) in the life sciences industry that is driven by its mission to unlock the power of data to improve health. Prognos Health’s exclusive, unique datasets unlock valuable insights in complex clinical populations across the entire commercial lifecycle, going beyond traditional RWD offerings. Prognos helps life sciences companies accelerate the development and delivery of innovative therapies and improve health outcomes by offering fully integrated and harmonized lab and health records on more than 325 million de-identified patients.
Introduction to Lab Data
Dr. Bhan, thanks for participating in the series! To begin, could you give us a quick overview of what we mean by “lab data?”
When we reference lab data at Prognos Health, we are referring to a comprehensive collection of test results from various diagnostic laboratories across the United States. This includes a wide variety of tests but we're particularly adept in areas like rare diseases and oncology, where specialized tests, like those from Next Generation Sequencing (NGS) labs, play a crucial role in diagnosing complex conditions.
Our data isn't limited to academic centers; it also comes from community hospitals and specialized clinics, offering a real-world snapshot of the treatment landscape for cancer and rare disease patients nationwide.
Why is lab data uniquely valuable for healthcare research? How does it compare to or complement other clinical and real-world data types, such as claims and EHR data?
Lab data stands out as a powerful tool in healthcare research due to its unique combination of objectivity, diagnostic power, and timeliness. Unlike subjective patient reports, lab tests provide quantifiable measures of health, offering a reliable and standardized way to assess biological functions and track disease progression. This objective nature plays a crucial role in diagnosing a wide range of diseases. Analyzing specific markers in blood, urine, or tissue samples can reveal underlying conditions early on, allowing for prompt intervention and improved patient outcomes. Furthermore, lab results are often available within 24 hours, significantly faster than EHR and claims data, which can take weeks or months to be finalized. This timeliness is crucial for researchers who need real-time insights into treatment effectiveness and disease progression.
Lab data complements other data sources like EHR and claims data to create a more holistic picture. When combined with EHR data, which provides clinical information about diagnoses, medications, and procedures, researchers can gain a more detailed understanding of a patient's condition, the treatments received, and how they responded. Integrating lab data with claims data, which focuses on billing information, reveals how often specific lab tests are ordered in real-world practice and how testing patterns relate to diagnoses and treatment costs.
In essence, lab data acts as the diagnostic workhorse, offering objective and timely results that are critical for early diagnosis, treatment monitoring, and, ultimately, improved patient care. EHR data, while valuable, can be subjective and incomplete, serving primarily as a record for healthcare providers. Claims data, on the other hand, reflects the business side of healthcare, focusing on billing information for diagnoses and treatments. By integrating all three data types, researchers gain a comprehensive understanding of patient health, disease progression, and treatment outcomes, ultimately leading to better healthcare strategies.
The Applications of Lab Data
Considering the growth of large-scale health databases like the UK Biobank and advancements in data analytics, how has de-identified lab data impacted healthcare research and personalized medicine?
Generally, de-identified lab data in combination with data analytics empowers researchers to find hidden patterns in lab results, leading to a deeper understanding of disease mechanisms and risk factors. A few specific benefits come to mind:
Lab data plays a role in the advancement of personalized medicine. By analyzing lab data alongside other patient information, researchers can tailor treatment plans for improved efficacy and reduced side effects.
Lab data has also enabled early disease detection. Large datasets can reveal subtle lab value changes that might precede symptoms, enabling early intervention and potentially preventing disease progression.
In the clinical R&D space, lab data aids in identifying drug targets and biomarkers for tracking new therapies, streamlining the drug development process.
Lastly, for public health researchers, analyzing large-scale lab data can inform targeted public health efforts by identifying areas with high disease prevalence or environmental risk factors.
Going a bit deeper, do any specific examples come to mind where lab data played an essential role in advancing healthcare research or discoveries?
Absolutely! Lab data has played a critical role in numerous breakthroughs across healthcare research. Two examples that come to mind are the development of precision medicine for multiple myeloma and the early detection of kidney disease.
Multiple myeloma is a blood cancer where specific genetic mutations and protein abnormalities in the bone marrow are crucial for diagnosis and treatment selection. Analyzing large datasets of lab results, including genetic tests and bone marrow biopsies, has been instrumental in identifying these key mutations and protein markers as well as understanding how these markers influence disease progression and response to treatment.
When researchers have access to de-identified lab data rich in genetic and protein biomarker information from a vast network of hospitals and labs, this data empowers that team to refine existing diagnostic tests and identify new ones and also to develop targeted therapies for specific patient subgroups based on their unique genetic and protein profiles.
Lab data also can be used to detect kidney disease before it progresses to later stages. Since kidney function tests are a cornerstone of early detection for chronic kidney disease, analyzing trends in blood tests like creatinine levels over time helps identify subtle changes that might indicate early-stage kidney dysfunction. Early detection is crucial for managing kidney disease and preventing complications. Large-scale lab data analysis can help refine risk prediction models and identify individuals who might benefit from preventive measures or early intervention strategies.
What trends are you witnessing with the application of de-identified lab data for research? Are there certain use cases that are more common or emerging?
The application of de-identified lab data for research is undergoing a fascinating evolution, with several key trends emerging.
Traditionally, research relied heavily on clinical trials. However, there's a growing emphasis on RWD, which includes de-identified lab data collected in real-world clinical settings. This data offers a more comprehensive picture of treatment effectiveness in everyday practice, complementing the controlled environment of clinical trials.
Of course, the vast amount of data within de-identified lab repositories is driving the application of AI and ML. These advanced analytics tools can identify complex patterns and relationships in the data, leading to new discoveries about disease mechanisms, treatment response variations, and potential drug targets.
Recognizing the value of larger datasets, researchers are advocating for better data-sharing practices and improved interoperability between different lab information systems. This allows for the creation of even more comprehensive de-identified lab data repositories for research purposes.
Lastly, as the use of de-identified lab data expands, there's a heightened focus on robust data governance practices, and ensuring patient privacy remains paramount. Consent management and anonymization techniques are being constantly refined to strike a balance between research needs and patient confidentiality.
As far as common use cases for lab data, three that stand out to me are:
- Personalized Medicine: Analyzing de-identified lab data alongside other patient information (e.g., genetics) allows researchers to identify subgroups with specific responses to treatments, paving the way for personalized medicine approaches.
- Drug Discovery and Development: De-identified lab data helps identify potential drug targets and biomarkers for tracking the effectiveness of new therapies during clinical trials.
- Public Health Initiatives: Analyzing large-scale lab data can help identify geographical areas with higher prevalence of specific diseases or uncover environmental factors that might contribute to certain health conditions. This information can be used to develop targeted public health initiatives and preventive measures.
The Challenges with Lab Data
Shifting to the potential challenges with using lab data: Sourcing, curating, and managing large volumes of lab data from multiple sources must be complex, especially given the importance of data provenance and data quality healthcare research and decision-making. Can you speak to these data challenges and how Prognos navigates them?
Sourcing, curating, and managing massive amounts of lab data from diverse sources is a significant challenge. The lab data is incredibly complex and messy, making the tasks of ensuring data provenance—knowing where each piece of data comes from—and maintaining high data quality absolutely critical for healthcare research and decision-making. Even the smallest inconsistencies can send us down the wrong path, leading to misleading results.
When we talk about the challenges of working with lab data, a few things come to mind. First, there's the issue of data heterogeneity. Lab data comes in all shapes and sizes, with formats and standards that can vary wildly from one lab to another, or between different healthcare systems. This diversity makes it quite a puzzle to fit all the pieces together. We have to consider data quality issues like missing points, coding inconsistencies, and the ever-present risk of errors creeping in during data entry. And let's not forget about the importance of keeping track of where each data point came from—the provenance—which is crucial for ensuring we can trust our research findings and replicate studies in the future.
On top of all this, there are significant privacy considerations. Even when working with de-identified data, we must ensure our anonymization practices are up to snuff to protect patient confidentiality without compromising the usefulness of the data.
At Prognos, we focus on standardizing and harmonizing data, transforming diverse data streams into a consistent and analyzable format. To tackle data quality head-on, we've established rigorous cleaning processes to fix errors and fill in the gaps, and we validate our data to ensure its accuracy. Keeping a detailed record of data provenance is also key for us; it helps researchers trace the data's origins and validate its reliability. And, of course, the privacy of patient data is paramount. We're committed to the highest standards of anonymization and secure data practices, all while staying aligned with the strictest data privacy regulations.
Double-clicking on the privacy angle, how do you balance the importance of data utility with the imperative of patient privacy and regulatory compliance?
Navigating the delicate balance between unlocking the power of data and protecting patient privacy is a critical challenge we face every day here at Prognos Health, especially as a US leader in handling de-identified lab data. Here’s how we tackle this.
We start by de-identifying data, stripping away direct identifiers like names and addresses, which lets researchers dig into trends without compromising patient confidentiality. We also set strict access controls, ensuring only trained researchers can access the data, with permissions tailored to their project needs. Data use agreements are in place to make sure researchers are clear on how to use the data responsibly.
Beyond just removing identifiers, we use advanced techniques like k-anonymity to further reduce re-identification risks. We believe in being transparent with patients about how their anonymized data is used for research, highlighting the benefits.
Complying with HIPAA regulations is critical for us. We regularly audit our practices to ensure we're not just compliant but are setting a high standard for data privacy and security.
This focus on a balanced approach allows us to leverage the valuable insights within de-identified lab data for research while safeguarding patient privacy and adhering to US data privacy regulations. It's a responsibility we take very seriously at Prognos Health.
Are there other major challenges with de-identified lab data that you’d like to highlight, along with any lessons learned on how to overcome them?
Beyond the core challenges of balancing data utility, privacy, and regulations, here are some other noteworthy hurdles associated with de-identified lab data, along with lessons learned for overcoming them.
For starters, there's the issue of data bias and generalizability. Sometimes, de-identified lab data skews towards certain demographics or comes mainly from urban hospitals, which can paint a misleading picture that doesn't quite match up with the broader population. The key lesson here is to be open about where the data's coming from and its limitations. It's crucial for researchers to keep an eye out for these biases and factor them into their analysis and interpretations.
Then, there's the challenge of knitting together data from a variety of sources. Labs and healthcare systems have their own ways of recording data, so you end up with this patchwork of formats and standards. At Prognos Health, we utilize data harmonization techniques to convert data from various sources into a consistent format. Standardizing data elements like test names, units, and reference ranges allows for seamless integration and analysis across different datasets.
And, of course, there’s data security. Even when data is anonymized, it's still a target for cyber threats. So, protecting this data is top of the list, with strong encryption, tight access controls, and regular security audits. This focus on data security fosters trust with researchers and the broader healthcare community.
Innovations and Future Opportunities
Let’s talk about future opportunities with lab data. When you think about how lab data is used for research today vs. how it could be used several years from now, what do you hope to see? In other words, what’s your vision for 2030?
By 2030, I see the landscape of lab data utilization for research undergoing a significant transformation. The fragmentation currently present in healthcare data will likely become a thing of the past. We'll see a seamless network that connects electronic health records, lab results, wearables, and other patient data sources into a comprehensive real-world data ecosystem. This will give us a more holistic view of patient health, enabling more comprehensive and generalizable studies.
I expect AI and machine learning to be at the forefront of this evolution, becoming even more sophisticated. These technologies will sift through massive datasets of lab data, not just identifying patterns but also predicting disease outbreaks, pinpointing high-risk patient populations, and uncovering novel drug targets with unparalleled accuracy. This could revolutionize preventative healthcare and personalized medicine approaches.
The democratization of lab data research is another development I anticipate. We'll likely see user-friendly platforms and standardized data formats that make lab data analysis accessible to a broader range of researchers. This will empower not just large institutions but also smaller research groups and individual scientists to contribute to groundbreaking discoveries.
I also foresee a focus on interoperability and privacy, with standardized data formats and secure, interoperable data-sharing platforms becoming the norm. This will streamline research collaboration and accelerate scientific progress. At the same time, robust privacy-preserving techniques like federated learning will ensure patient data remains secure throughout the analysis process.
Integration with genomics and microbiome data is something I'm particularly excited about. We'll be able to seamlessly integrate lab data with an individual's genetic makeup and microbiome analysis. This comprehensive approach to health could lead to the development of truly personalized treatments and preventive measures tailored to an individual's unique biology.
How is Prognos playing a part in achieving that vision?
The team at Prognos Health is very passionate about achieving the future vision where lab data becomes a true transformative force in research. When we look at our BHAG of 20 billion health insights delivered by 2050 here’s some of what we are focused on:
Firstly, we're dedicated to ensuring data quality and standardization. By meticulously cleaning and harmonizing our de-identified lab data, we make it simpler for researchers to blend data from various sources, enabling more thorough analyses. This effort is key to creating the seamless real-world data ecosystems we envision for healthcare research.
Collaboration and the spirit of open science are also central to our mission. We're building bridges with researchers and institutions by providing access to our high-quality, de-identified lab data for legitimate research. This approach speeds up scientific progress and supports the vision of democratizing lab data research, opening doors for a broader array of researchers to make significant contributions.
Privacy is a non-negotiable aspect of our work. We're exploring cutting-edge anonymization techniques and the potential use of federated learning to safeguard patient privacy. This aligns with the envisioned future where robust security measures support efficient data sharing for research.
We're also investing heavily in advanced analytics, evaluating and integrating AI and machine learning tools into our analysis processes. This investment is laying the groundwork for future breakthroughs in AI-driven disease prediction and the development of personalized medicine, which will lead to more precise and effective treatments.
Lastly, keeping abreast of changes in data privacy regulations and technological advances is crucial for us. We're committed to staying informed and adaptable, ensuring our practices are compliant and supportive of a future where data interoperability and responsible utilization are standard in healthcare research.
We are laying the groundwork for the future vision of transformative lab data utilization. Our commitment to data quality, collaboration, privacy, and advanced analytics positions us as a key player in accelerating research progress and ultimately transforming healthcare for the better.
Thank you for your time today. Where can our readers go to learn more about lab data, precision medicine research, and Prognos?
Visit us on our website at www.prognoshealth.com or email us directly at marketing@prognoshealth.com.